Mar 18 2013
Urs Wilke of the EPFL Solar Energy and Building Physics Laboratory has successfully defended his PhD thesis on the probabilistic bottom-up modelling of occupancy and activities to predict electricity demand in residential buildings.
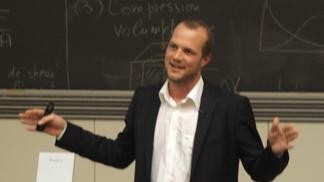
To reduce energy demand in buildings and to expand the use of renewable energies, it is crucial to develop strategies and solutions that optimally exhaust the possibilities of improving the efficiency of these systems. Dynamic simulation models are increasingly used to gain a more precise understanding of the underlying processes of energy flows in buildings. However, many of the fundamental phenomena are still not suffciently understood, resulting in potentially significant errors.
The description of occupants' behaviour in particular still leaves substantial room for improvement in the simulations; there is a lack of comprehensive and validated stochastic models predicting residential occupancy and activities, as well as their variations between individuals and households. However, the stochastic nature of residential behaviour is central regarding uncertainties in residential buildings' energy demand.
Urs Wilke's PhD thesis develops adequate bottom-up models to predict time-dependent residential occupancy and activities, as well as household appliance ownership as a function of individual characteristics, and further proposes an innovative approach to relate the use of electrical appliances to the activities performed. The models are calibrated with detailed survey statistics of individuals' time use, as well as households' appliance ownership and appliances' power consumption.
The versatile approach adopted in this thesis results in a valuable methodology to predict electricity demand profiles in various scenarios, which can be used, for instance, to assess the reliability of decentralised power generation infrastructures, which are subject to considerable fluctuations.